A panel of three experts explained how, when designed effectively, studies can harness real-world data for vital insights into patient populations in Real-World Data in Diabetes Research—Promise or Peril?
The session can be viewed by registered meeting participants at ADA2023.org. If you haven’t registered for the 83rd Scientific Sessions, register today to access the valuable meeting content through August 28.
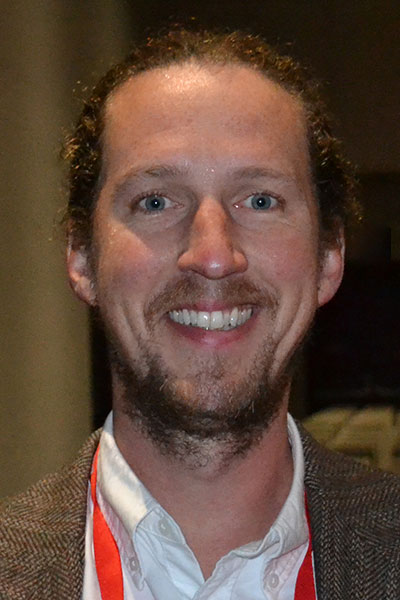
The U.S. Food and Drug Administration (FDA) defines real-world data as information relating to patient health status and/or the delivery of health care routinely collected from sources such as electronic health records (EHR), medical claims, and product or disease registries.
Alan Kinlaw, PhD, Assistant Professor in the Division of Pharmaceutical Outcomes and Policy, University of North Carolina at Chapel Hill, clarified that real-world data is edited and organized into a derived analytical dataset rather than raw data.
To ensure data validity, he recommends first finding out why and how the data were made. Understanding the context from which the data originated can help researchers determine whether they should use it in their own work. Dr. Kinlaw also emphasized the necessity of engaging with a multidisciplinary team and framework from his own research experience as an epidemiologist.
He highlighted the value of design diagrams, calling them “the most important tool” for researchers.
“This helps you disengage from conceptions that you might have about temporality and makes you say exactly where you’re measuring and when you’re measuring,” he explained.
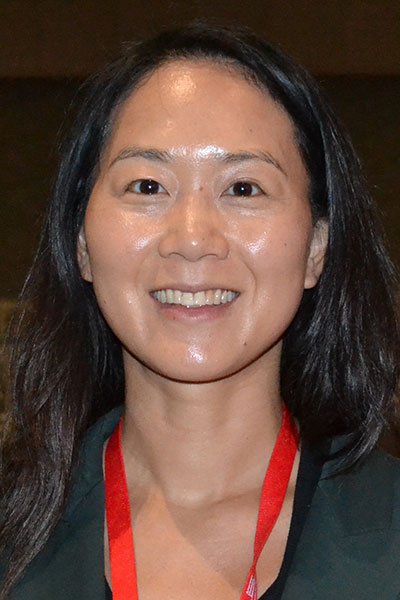
Shirley Wang, PhD, Associate Professor of Medicine, Harvard University, and Kristi Reynolds, PhD, MPH, FAHA, FACE, Director of Epidemiologic Research for the Department of Research & Evaluation for Kaiser Permanente, shared case studies to illustrate the effectiveness of real-world data.
A 2020 study asserted that randomized clinical trials (RCTs) were superior to database studies utilizing real-world data. Dr. Wang’s recently published research rebutted this assertion, concluding that non-randomized real-world evidence studies came to similar conclusions about a drug’s treatment effect as randomized trials when data was fit-for-purpose and utilized proper design and analysis. Dr. Wang emulated the design of 30 completed and two ongoing RCTs of medications with non-randomized real-world data.
“The real benefit of database studies comes from being able to generate relevant clinical insights about questions that, for a variety of reasons, that trial cannot be conducted,” she said.
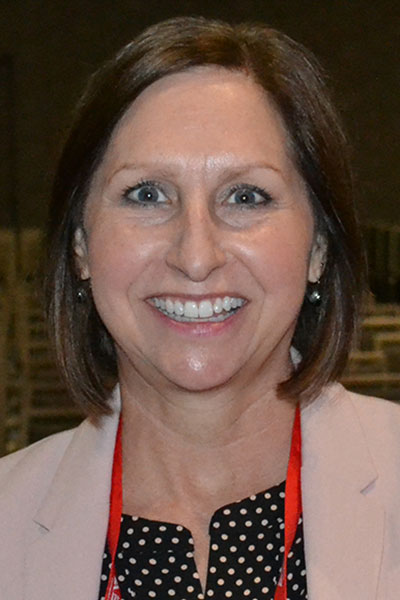
Dr. Reynolds discussed her experience working with the Diabetes by Type in Children, Adolescents, and Young Adults (DiCAYA) Network study. Researchers are using EHR data to measure the prevalence of incidence of diabetes in people younger than 18 years. She said EHR-based surveillance holds promise while being relatively low-cost.
The research group first developed EHR-based computable phenotypes for accurate identification of the incidence and prevalence of type 1 and type 2 diabetes among youth and young adults in the U.S. These can provide estimates by diabetes type, age, sex, race, and geographic area while evaluating key surveillance attributes.
Dr. Reynolds outlined various opportunities for the use of real-world EHR data in research. Due to the widespread adoption of EHR systems nationwide, the data are generalizable to a variety of settings. It also allows researchers to obtain more efficient and timely information than traditional disease surveillance resources. Additionally, investigators can expand their surveillance to examine long-term trends or other medical conditions.
“We are also able to test different approaches to bias, and adjust and improve estimation to geographic populations,” she explained.
The main challenges of working with real-world EHR data included strict policies around data sharing, creating common data models across systems, data quality concerns, and the potential for selection bias of non-representative patient populations.
Dr. Reynolds explained that other organizations will get the chance to build on the DiCAYA Network’s framework.
“The network will disseminate the EHR-based surveillance methodology that could be broadly adopted because it’s a centrist representative mix of health systems, including membership-based health systems, the practice-based health systems, and geographically-based systems,” she said.